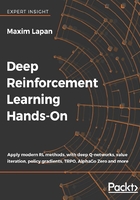
Tensors
A tensor is the fundamental building block of all DL toolkits. The name sounds cool and mystic, but the underlying idea is that a tensor is a multi-dimensional array. One single number is like a point, which is zero-dimensional, while a vector is one-dimensional like a line segment, and a matrix is a two-dimensional object. Three-dimensional number collections can be represented by a parallelepiped of numbers, but don't have a separate name in the same way as matrix. We can keep this term for collections of higher dimensions, which are named multi-dimensional matrices or tensors.

Figure 1: Going from a single number to an n-dimension tensor
Creation of tensors
If you're familiar with the NumPy library (and you should be), then you already know that its central purpose is the handling of multi-dimensional arrays in a generic way. In NumPy, such arrays aren't called tensors, but, in fact, they are tensors. Tensors are used very widely in scientific computations, as generic storage for data. For example, a color image could be encoded as a 3D tensor with dimensions of width, height, and color plane.
Apart from dimensions, a tensor is characterized by the type of its elements. There are eight types supported by PyTorch: three float types (16-bit, 32-bit, and 64-bit) and five integer types (8-bit signed, 8-bit unsigned, 16-bit, 32-bit, and 64-bit). Tensors of different types are represented by different classes, with the most commonly used being torch.FloatTensor
(corresponding to a 32-bit float), torch.ByteTensor
(an 8-bit unsigned integer), and torch.LongTensor
(a 64-bit signed integer). The rest can be found in the documentation.
There are three ways to create a tensor in PyTorch:
- By calling a constructor of the required type.
- By converting a NumPy array or a Python list into a tensor. In this case, the type will be taken from the array's type.
- By asking PyTorch to create a tensor with specific data for you. For example, you can use the
torch.zeros()
function to create a tensor filled with zero values.
To give you examples of these methods, let's look at a simple session:
>>> import torch >>> import numpy as np >>> a = torch.FloatTensor(3, 2) >>> a tensor([[ 4.1521e+09, 4.5796e-41], [ 1.9949e-20, 3.0774e-41], [ 4.4842e-44, 0.0000e+00]])
Here, we imported both PyTorch and NumPy and created an uninitialized tensor of size 3 × 2. By default, PyTorch allocates memory for the tensor, but doesn't initialize it with anything. To clear the tensor's content, we need to use its operation:
>>> a.zero_() tensor([[ 0., 0.], [ 0., 0.], [ 0., 0.]])
There are two types of operation for tensors: inplace and functional. Inplace operations have an underscore appended to their name and operate on the tensor's content. After this, the object itself is returned. The functional equivalent creates a copy of the tensor with the performed modification, leaving the original tensor untouched. Inplace operations are usually more efficient from a performance and memory point of view.
Another way to create a tensor by its constructor is to provide a Python iterable (for example, a list or tuple), which will be used as the contents of the newly created tensor:
>>> torch.FloatTensor([[1,2,3],[3,2,1]]) tensor([[ 1., 2., 3.], [ 3., 2., 1.]])
Here we are creating the same zero object using NumPy:
>>> n = np.zeros(shape=(3, 2)) >>> n array([[ 0., 0.], [ 0., 0.], [ 0., 0.]]) >>> b = torch.tensor(n) >>> b tensor([[ 0., 0.], [ 0., 0.], [ 0., 0.]], dtype=torch.float64)
The torch.tensor
method accepts the NumPy array as an argument and creates a tensor of appropriate shape from it. In the preceding example, we created a NumPy array initialized by zeros, which created a double (64-bit float) array by default. So, the resulting tensor has the DoubleTensor
type (which is shown in the preceding example with the dtype
value). Usually, in DL, double precision is not required and it adds an extra memory and performance overhead. The common practice is to use the 32-bit float type, or even the 16-bit float type, which is more than enough. To create such a tensor, you need to specify explicitly the type of NumPy array:
>>> n = np.zeros(shape=(3, 2), dtype=np.float32) >>> torch.tensor(n) tensor([[ 0., 0.], [ 0., 0.], [ 0., 0.]])
As an option, the type of the desired tensor could be provided to the torch.tensor
function in the dtype
argument. However, be careful, since this argument expects to get a PyTorch type specification, not the NumPy one. PyTorch types are kept in the torch
package, for example, torch.float32
, torch.uint8
.
>>> n = np.zeros(shape=(3,2)) >>> torch.tensor(n, dtype=torch.float32) tensor([[ 0., 0.], [ 0., 0.], [ 0., 0.]])
Compatibility note: The torch.tensor()
method and explicit PyTorch type specification were added in the 0.4.0 release, and this is a step toward simplification of tensor creation. In previous versions, the torch.from_numpy()
function was a recommended way to convert NumPy arrays, but it had issues with handling the combination of the Python list and NumPy arrays. This from_numpy()
function is still present for backward compatibility, but it is deprecated in favor of the more flexible torch.tensor()
method.
Scalar tensors
Since the 0.4.0 release, PyTorch supports zero-dimensional tensors that correspond to scalar values (on the left of Figure 1). Such tensors can be a result of some operations, such as summing all values in a tensor. Earlier, such cases were handled by the creation of a one-dimension (vector) tensor with single dimension equal to one. This solution worked, but wasn't very simple, as extra indexation was needed to access the value.
Now zero-dimension tensors are natively supported and returned by the appropriate functions and can be created by the torch.tensor()
function. To access the actual Python value of such a tensor, they have the special item()
method:
>>> a = torch.tensor([1,2,3]) >>> a tensor([ 1, 2, 3]) >>> s = a.sum() >>> s tensor(6) >>> s.item() 6 >>> torch.tensor(1) tensor(1)
Tensor operations
There are lots of operations that you can perform on tensors, and there are too many to list them all. Usually, it's enough to search in the PyTorch documentation at http://pytorch.org/docs/. Here we need to mention that besides the inplace and functional variants we already discussed (that is, with and without underscore, like zero()
and zero_()
), there are two places to look for operations: the torch
package and the tensor class. In the first case, the function usually accepts the tensor as an argument. In the second, it operates on the called tensor.
Most of the time, tensor operations are trying to correspond to their NumPy equivalent, so if there is some not-very-specialized function in NumPy, then there is a good chance that PyTorch will also have it. Examples are torch.stack()
, torch.transpose()
, and torch.cat()
.
GPU tensors
PyTorch transparently supports CUDA GPUs, which means that all operations have two versions—CPU and GPU—which are automatically selected. The decision is made based on the type of tensors that you are operating on. Every tensor type that we mentioned is for CPU and has its GPU equivalent. The only difference is that GPU tensors reside in the torch.cuda
package, instead of just torch
. For example, torch.FloatTensor
is a 32-bit float tensor which resides in CPU memory, but torch.cuda.FloatTensor
is its GPU counterpart. To convert from CPU to GPU, there is a tensor method, to(device)
, which creates a copy of the tensor to a specified device (which could be CPU or GPU). If the tensor is already on the device, nothing happens and the original tensor will be returned. Device type can be specified in different ways. First of all, you can just pass a string name of the device, which is "cpu" for CPU memory or "cuda" for GPU. A GPU device could have an optional device index specified after the colon, for example, the second GPU card in the system could be addressed by "cuda:1" (index is zero-based).
Another slightly more efficient way to specify a device in the to()
method is using the torch.device
class, which accepts the device name and optional index. For accessing the device that your tensor is currently residing in, it has a device
property.
>>> a = torch.FloatTensor([2,3]) >>> a tensor([ 2., 3.]) >>> ca = a.cuda(); ca tensor([ 2., 3.], device='cuda:0')
Here, we created a tensor on CPU, then copied it to GPU memory. Both copies could be used in computations and all GPU-specific machinery is transparent to the user:
>>> a + 1 tensor([ 3., 4.]) >>> ca + 1 tensor([ 3., 4.], device='cuda:0') >>> ca.device device(type='cuda', index=0)
Compatibility note: The to()
method and torch.device
class were introduced in 0.4.0. In previous versions, copying between CPU and GPU was performed by separate tensor methods, cpu()
and cuda()
, respectively, which required adding the extra lines of code to explicitly convert tensors into their CUDA versions. In the latest version, you can create a desired torch.device
object in the beginning of the program and use to(device)
on every tensor you're creating. The old methods, cpu()
and cuda()
in the tensor are still present, but deprecated.