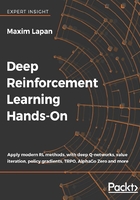
Monitoring with TensorBoard
If you have ever tried to train a NN on your own, then you may know how painful and uncertain it can be. I'm not talking about following the existing tutorials and demos, when all hyperparameters are already tuned for you, but about taking some data and creating something from scratch. Even with modern DL high-level toolkits, where all best practices such as proper weights initialization and optimizers' betas, gammas, and other options are set to sane defaults, and tons of other stuff is hidden under the hood, there are still lots of decisions that you can make, hence lots of things could go wrong. As a result, your network almost never works from the first run and this is something that you should get used to.
Of course, with practice and experience, you'll develop a strong intuition about the possible causes of problems, but intuition needs input data about what's going on inside your network. So you need to be able to peek inside your training process somehow and observe its dynamics. Even small networks (such as tiny MNIST tutorial networks) could have hundreds of thousands of parameters with quite nonlinear training dynamics. DL practitioners have developed a list of things that you should observe during your training, which usually includes the following:
- Loss value, which normally consists of several components like base loss and regularization losses. You should monitor both total loss and inpidual components over time.
- Results of validation on training and test sets.
- Statistics about gradients and weights.
- Learning rates and other hyperparameters, if they are adjusted over time.
The list could be much longer and include domain-specific metrics, such as word embeddings' projections, audio samples, and images generated by GAN. You also may want to monitor values related to training speed, like how long an epoch takes, to see the effect of your optimizations or problems with hardware.
To make a long story short, you need a generic solution to track lots of values over time and represent them for analysis, preferably developed specially for DL (just imagine looking at such statistics in an Excel spreadsheet). Luckily, such tools exist.
TensorBoard 101
In fact, at the time of writing, there are not many alternatives to choose from, especially open source and generic ones. From the first public version, TensorFlow included a special tool called TensorBoard, developed to solve the problem we are talking about: how to observe and analyze various NN characteristics over training. TensorBoard is a powerful, generic solution with a large community and it looks quite pretty:

Figure 4: The TensorBoard web interface
From the architecture point of view, TensorBoard is a Python web service which you can start on your computer, passing it the directory where your training process will save values to be analyzed. Then you point your browser to TensorBoard's port (usually 6006
), and it shows you an interactive web interface with values updated in real-time. It's nice and convenient, especially when your training is performed on a remote machine somewhere in the cloud.
Originally, TensorBoard was deployed as a part of TensorFlow, but recently, it has been moved to a separate project (it's still being maintained by Google) and has its own package name. However, TensorBoard still uses the TensorFlow data format, so to be able to write training statistics from PyTorch optimization, you'll need both the tensorflow
and tensorflow-tensorboard
packages installed. As TensorFlow depends on TensorBoard, to install both, you need to run pip install tensorflow
in your virtual environment.
In theory, this is all you need to start monitoring your networks, as the tensorflow
package provides you with classes to write the data that TensorBoard will be able to read. However, it's not very practical, as those classes are very low level. To overcome this, there are several third-party open-source libraries that provide a convenient high-level interface. One of my favorites, which is used in this book, is tensorboard-pytorch
(https://github.com/lanpa/tensorboard-pytorch). It can be installed with pip install tensorboard-pytorch
.
Plotting stuff
To give you an impression of how simple tensorboard-pytorch
is, let's consider a small example that is not related to NNs, but is just about writing stuff into TensorBoard (the full example code is in Chapter03/02_tensorboard.py
).
import math from tensorboardX import SummaryWriter if __name__ == "__main__": writer = SummaryWriter() funcs = {"sin": math.sin, "cos": math.cos, "tan": math.tan}
We import the required packages, create a writer of data, and define functions that we're going to visualize. By default, SummaryWriter
will create a unique directory under the runs
directory for every launch, to be able to compare different launches of training. Names of the new directory include the current date and time, and hostname. To override this, you can pass the log_dir
argument to SummaryWriter
. You also can add a suffix to the name of the directory by passing a comment option, for example to capture different experiments' semantics, such as dropout=0.3
or strong_regularisation
for angle in range(-360, 360): angle_rad = angle * math.pi / 180 for name, fun in funcs.items(): val = fun(angle_rad) writer.add_scalar(name, val, angle) writer.close()
Here, we loop over angle ranges in degrees, convert them into radians, and calculate our functions' values. Every value is being added to the writer using the add_scalar
function, which takes three arguments: the name of the parameter, its value, and the current iteration (which has to be an integer).
The last thing we need to do after the loop is to close the writer. Note that the writer does a periodical flush (by default, every two minutes), so even in the case of a lengthy optimization process, you still will see your values.
The result of running this will be zero output on the console, but you will see a new directory created inside the runs
directory with a single file. To look at the result, we need to start TensorBoard:
rl_book_samples/Chapter03$ tensorboard --logdir runs --host localhost TensorBoard 0.1.7 at http://localhost:6006 (Press CTRL+C to quit)
Now you can open http://localhost:6006
in your browser to see something like this:

Figure 5: Plots produced by the example
The graphs are interactive, so you can hover over them with your mouse to see the actual values and select regions to zoom into details. To zoom out, double-click inside the graph. If you run your program several times, then you will see several items in the "runs" list on the left, which can be enabled and disabled in any combinations, allowing you to compare the dynamics of several optimizations. TensorBoard allows you to analyze not only scalar values but also images, audio, text data, and embeddings, and it can even show you the structure of your network. Refer to the documentation of tensorboard-pytorch
and tensorboard
for all those features.
Now it's time to unite everything you learned in this chapter and look at a real NN optimization problem using PyTorch.